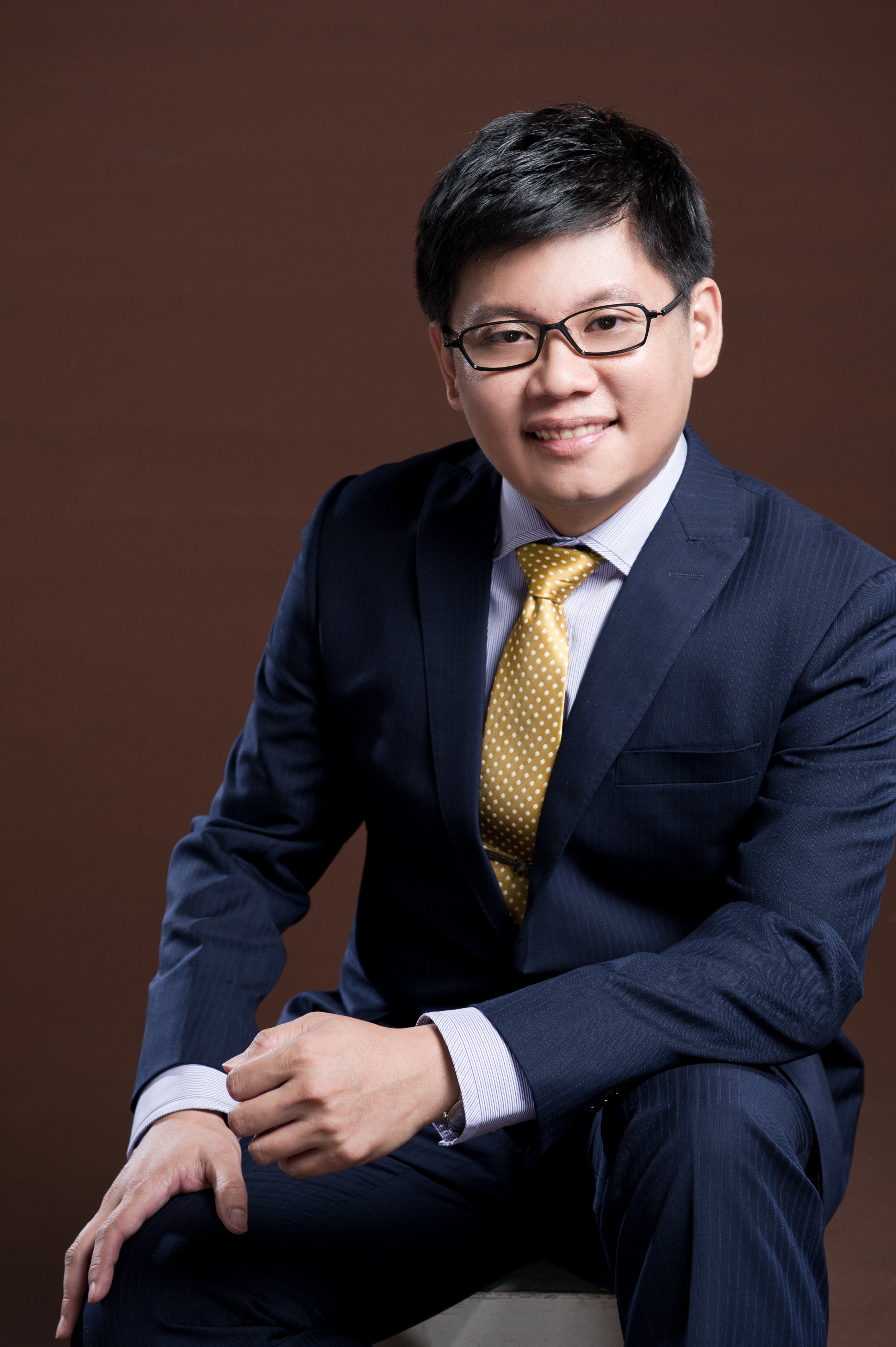
Understanding Deep Neural Networks
- Biography
- Speech
- Slido
Biography
Shou-de Lin is currently a full professor in the CSIE department of National Taiwan University. He holds a BS degree in EE department from National Taiwan University, an MS-EE degree from the University of Michigan, an MS degree in Computational Linguistics and PhD in Computer Science both from the University of Southern California. He leads the Machine Discovery and Social Network Mining Lab in NTU. Before joining NTU, he was a post-doctoral research fellow at the Los Alamos National Lab. Prof. Lin’s research includes the areas of machine learning and data mining, social network analysis, and natural language processing. His international recognition includes the best paper award in IEEE Web Intelligent conference 2003, Google Research Award in 2007, Microsoft research award in 2008, 2015, 2016 merit paper award in TAAI 2010, 2014, 2016, best paper award in ASONAM 2011, US Aerospace AFOSR/AOARD research award winner for 5 years. He is the all-time winners in ACM KDD Cup, leading or co-leading the NTU team to win 5 championships. He also leads a team to win WSDM Cup 2016. He has served as the senior PC for SIGKDD and area chair for ACL. He is currently the associate editor for International Journal on Social Network Mining, Journal of Information Science and Engineering, and International Journal of Computational Linguistics and Chinese Language Processing. He is also a freelance writer for Scientific American.
Speech
Artificial Intelligence has become overwhelming popular in recent years thanks to availability of big data and the advance of machine learning based models. Among them, solutions based on the Deep Neural Network (or DNN) have produced tremendous success in various applications including computer vision, natural language processing, etc. Nevertheless, one common concern for DNN-based solutions is that they are generally very complicated and can hardly be understood by human beings. This talk focuses on a specific type of deep neural networks called recurrent neural networks (RNN). It will not only demonstrate the power of an RNN model to learn from implicit information but also explain why the overwhelming performance can be achieved given its architecture.