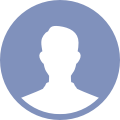
Mr. Gajendra Deshpande holds a masters degree in Computer Science and Engineering and working as Assistant Professor at the Department of Computer Science and Engineering, KLS Gogte Institute of Technology, Belagavi, Karnataka, India. He has a teaching experience of 12 years and a Linux and Network Administration experience of one year. Under his mentor-ship teams have won Smart India Hackathon 2018 and Smart India Hackathon 2019. He is the Technical Director for Sestoauto Networks Pvt. Ltd. and Founder of Thingsvalley. His areas of Interest include Programming, Web Designing, Cyber Security, Artificial Intelligence, Machine Learning, Brain-Computer Interface, Internet of Things, and Virtual Reality. He has presented papers at NIT Goa, Scipy India 2017 IIT Bombay, JuliaCon 2018 London, Scipy India 2018 IIT Bombay, Scipy 2019 USA, and PyCon FR 2019, Bordeaux, France.